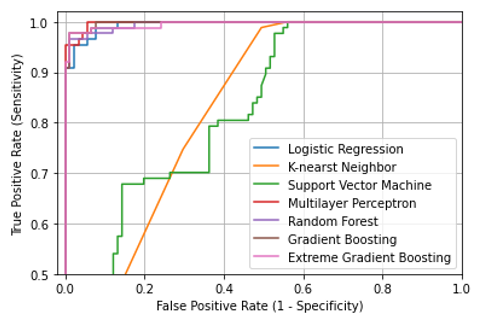
Classification of IGF1R ligand compounds for Identification of herbal extracts using extreme gradient boosting
Abstract
Keywords
Full Text:
PDFReferences
A. Sapra and P. Bhandari, Diabetes Mellitus. StatPearls Publishing, Treasure Island (FL), 2019.
J. B. Cole and J. C. Florez, “Genetics of diabetes mellitus and diabetes complications,†Nat. Rev. Nephrol. 2020 167, vol. 16, no. 7, pp. 377–390, May 2020, doi: 10.1038/s41581-020-0278-5.
O. O. Oguntibeju, “Type 2 diabetes mellitus, oxidative stress and inflammation: examining the links,†Int. J. Physiol. Pathophysiol. Pharmacol., vol. 11, no. 3, p. 45, 2019, [Online]. Available: /pmc/articles/PMC6628012/.
H. D. McIntyre, P. Catalano, C. Zhang, G. Desoye, E. R. Mathiesen, and P. Damm, “Gestational diabetes mellitus,†Nat. Rev. Dis. Prim. 2019 51, vol. 5, no. 1, pp. 1–19, Jul. 2019, doi: 10.1038/s41572-019-0098-8.
E. N. Gonc et al., “Genetic IGF1R defects: new cases expand the spectrum of clinical features,†J. Endocrinol. Investig. 2020 4312, vol. 43, no. 12, pp. 1739–1748, Apr. 2020, doi: 10.1007/S40618-020-01264-Y.
M. Hamim, Z. Al, M. I. Irawan, N. Nyoman, and T. Puspaningsih, “Prediction insulin-protein interactions associated based on ontology genes using extreme gradient boosting and centrality method,†Kinet. Game Technol. Inf. Syst. Comput. Network, Comput. Electron. Contr, vol. 4, no. 5, pp. 253–262, 2020, doi: https://doi.org/10.22219/kinetik.v5i4.107.
Y. Khajebishak, L. Payahoo, M. Alivand, and B. Alipour, “Punicic acid: A potential compound of pomegranate seed oil in Type 2 diabetes mellitus management,†J. Cell. Physiol., vol. 234, no. 3, pp. 2112–2120, Mar. 2019, doi: 10.1002/JCP.27556.
K. A. Carpenter and X. Huang, “Machine Learning-based Virtual Screening and Its Applications to Alzheimer’s Drug Discovery: A Review,†Curr. Pharm. Des., vol. 24, no. 28, pp. 3347–3358, Dec. 2018, doi: 10.2174/1381612824666180607124038.
Y. Peng and M. H. Nagata, “An empirical overview of nonlinearity and overfitting in machine learning using COVID-19 data,†Chaos, Solitons & Fractals, vol. 139, p. 110055, Oct. 2020, doi: 10.1016/J.CHAOS.2020.110055.
Y. Zhou et al., “Quantitative Structure-Activity Relationship (QSAR) Model for the Severity Prediction of Drug-Induced Rhabdomyolysis by Using Random Forest,†Chem. Res. Toxicol., vol. 34, no. 2, pp. 514–521, Feb. 2021, doi: 10.1021/ACS.CHEMRESTOX.0C00347/SUPPL_FILE/TX0C00347_SI_001.ZIP.
A. Capecchi, D. Probst, and J. L. Reymond, “One molecular fingerprint to rule them all: Drugs, biomolecules, and the metabolome,†J. Cheminform., vol. 12, no. 1, pp. 1–15, Jun. 2020, doi: 10.1186/S13321-020-00445-4/FIGURES/8.
M. M. Mysinger, M. Carchia, J. J. Irwin, and B. K. Shoichet, “Directory of useful decoys, enhanced (DUD-E): Better ligands and decoys for better benchmarking,†J. Med. Chem., vol. 55, no. 14, pp. 6582–6594, 2012, doi: 10.1021/jm300687e.
S. Kim et al., “PubChem in 2021: new data content and improved web interfaces,†Nucleic Acids Res., vol. 49, no. D1, pp. D1388–D1395, Jan. 2021, doi: 10.1093/NAR/GKAA971.
M. Bagherian, E. Sabeti, K. Wang, M. A. Sartor, Z. Nikolovska-Coleska, and K. Najarian, “Machine learning approaches and databases for prediction of drug–target interaction: a survey paper,†Brief. Bioinform., vol. 22, no. 1, pp. 247–269, Jan. 2021, doi: 10.1093/BIB/BBZ157.
Y. Y. S. Rahayu, T. Araki, and D. Rosleine, “Factors affecting the use of herbal medicines in the universal health coverage system in Indonesia,†J. Ethnopharmacol., vol. 260, p. 112974, Oct. 2020, doi: 10.1016/J.JEP.2020.112974.
P. I. Koukos, M. Réau, and A. M. J. J. Bonvin, “Shape-Restrained Modeling of Protein-Small-Molecule Complexes with High Ambiguity Driven DOCKing,†J. Chem. Inf. Model., vol. 61, no. 9, pp. 4807–4818, Sep. 2021, doi: 10.1021/ACS.JCIM.1C00796/SUPPL_FILE/CI1C00796_SI_002.XLSX.
N. R. Das, S. P. Mishra, and P. G. R. Achary, “Evaluation of molecular structure based descriptors for the prediction of pEC50(M) for the selective adenosine A2A Receptor,†J. Mol. Struct., vol. 1232, p. 130080, May 2021, doi: 10.1016/J.MOLSTRUC.2021.130080.
H. Kuswanto, R. Y. Nurhidayah, and H. Ohwada, “Comparison of Feature Selection Methods to Classify Inhibitors in DUD-E Database,†in Procedia Computer Science, Jan. 2018, vol. 144, pp. 194–202, doi: 10.1016/j.procs.2018.10.519.
A. Salazar, L. Vergara, and G. Safont, “Generative Adversarial Networks and Markov Random Fields for oversampling very small training sets,†Expert Syst. Appl., vol. 163, p. 113819, Jan. 2021, doi: 10.1016/J.ESWA.2020.113819.
A. Fitriawan, I. Wasito, A. F. Syafiandini, M. Amien, and A. Yanuar, “Deep belief networks using hybrid fingerprint feature for virtual screening of drug design,†in 2016 International Conference on Advanced Computer Science and Information Systems, ICACSIS 2016, Mar. 2017, pp. 417–420, doi: 10.1109/ICACSIS.2016.7872737.
A. Capecchi, M. Awale, D. Probst, and J. Reymond, “PubChem and ChEMBL beyond Lipinski,†Mol. Inform., vol. 38, no. 5, p. 1900016, May 2019, doi: 10.1002/minf.201900016.
K. Dührkop et al., “SIRIUS 4: a rapid tool for turning tandem mass spectra into metabolite structure information,†Nat. Methods, vol. 16, no. 4, pp. 299–302, Apr. 2019, doi: 10.1038/S41592-019-0344-8.
S. Kim, P. A. Thiessen, E. E. Bolton, and S. H. Bryant, “PUG-SOAP and PUG-REST: Web services for programmatic access to chemical information in PubChem,†Nucleic Acids Res., vol. 43, no. W1, pp. W605–W611, 2015, doi: 10.1093/NAR/GKV396.
T. Chen and C. Guestrin, “XGBoost: A scalable tree boosting system,†in Proceedings of the ACM SIGKDD International Conference on Knowledge Discovery and Data Mining, 2016, vol. 13-17-Augu, pp. 785–794, doi: 10.1145/2939672.2939785.
M. Rahman, Y. Cao, X. Sun, B. Li, and Y. Hao, “Deep pre-trained networks as a feature extractor with XGBoost to detect tuberculosis from chest X-ray,†Comput. Electr. Eng., vol. 93, p. 107252, Jul. 2021, doi: 10.1016/J.COMPELECENG.2021.107252.
M. R. Mohammadi et al., “Modeling hydrogen solubility in hydrocarbons using extreme gradient boosting and equations of state,†Sci. Reports 2021 111, vol. 11, no. 1, pp. 1–20, Sep. 2021, doi: 10.1038/s41598-021-97131-8.
R. R. Syahdi, J. T. Iqbal, A. Munim, and A. Yanuar, “HerbalDB 2.0: Optimization of construction of three-dimensional chemical compound structures to update Indonesian medicinal plant database,†Pharmacogn. J., vol. 11, no. 6, pp. 1189–1194, Jan. 2019, doi: 10.5530/PJ.2019.11.184.
S. Bagui and K. Li, “Resampling imbalanced data for network intrusion detection datasets,†J. Big Data, vol. 8, no. 1, pp. 1–41, Dec. 2021, doi: 10.1186/S40537-020-00390-X/TABLES/49.
R. Singh et al., “Classification of beta-site amyloid precursor protein cleaving enzyme 1 inhibitors by using machine learning methods,†Chem. Biol. Drug Des., vol. 98, no. 6, pp. 1079–1097, Dec. 2021, doi: 10.1111/CBDD.13965.
R. Couronné, P. Probst, and A. L. Boulesteix, “Random forest versus logistic regression: A large-scale benchmark experiment,†BMC Bioinformatics, vol. 19, no. 1, 2018, doi: 10.1186/s12859-018-2264-5.
N. K. Hepler, A. Bowman, R. E. Carey, and D. J. Cosgrove, “Expansin gene loss is a common occurrence during adaptation to an aquatic environment,†Plant J., vol. 101, no. 3, pp. 666–680, Feb. 2020, doi: 10.1111/TPJ.14572.
DOI: http://dx.doi.org/10.26555/jifo.v16i3.a23286
Refbacks
- There are currently no refbacks.
Copyright (c) 2022 Mohammad Hamim Zajuli Al Faroby, Siti Amiroch, Bernadus Anggo Seno Aji, Avriono Aritonang

This work is licensed under a Creative Commons Attribution-ShareAlike 4.0 International License.
____________________________________
JURNAL INFORMATIKA
ISSN :Â 1978-0524 (print) | 2528-6374 (online)
This work is licensed under a Creative Commons Attribution-ShareAlike 4.0 International License.